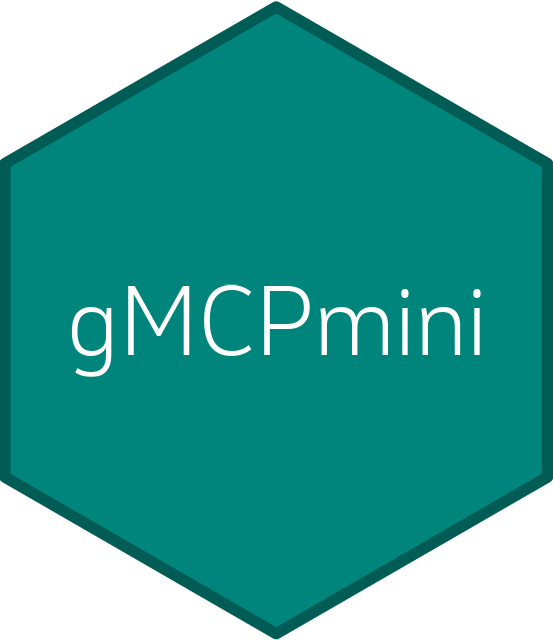
Simultaneous confidence intervals for sequentially rejective multiple test procedures
Source:R/graphMCP.R
simConfint.Rd
Calculates simultaneous confidence intervals for sequentially rejective multiple test procedures.
Usage
simConfint(
object,
pvalues,
confint,
alternative = c("less", "greater"),
estimates,
df,
alpha = 0.05,
mu = 0
)
Arguments
- object
A graph of class
graphMCP
.- pvalues
A numeric vector specifying the p-values for the sequentially rejective MTP.
- confint
One of the following: A character string
"normal"
,"t"
or a function that calculates the confidence intervals. Ifconfint=="t"
the parameterdf
must be specified. Ifconfint
is a function it must be of signature("character", "numeric")
, where the first parameter is the hypothesis name and the second the marginal confidence level (see examples).- alternative
A character string specifying the alternative hypothesis, must be "greater" or "less".
- estimates
Point estimates for the parameters of interest.
- df
Degree of freedom as numeric.
- alpha
The overall alpha level as numeric scalar.
- mu
The numerical parameter vector under null hypothesis.
Value
A matrix with columns giving lower confidence limits, point estimates and upper confidence limits for each parameter. These will be labeled as "lower bound", "estimate" and "upper bound".
(1-level)/2 in % (by default 2.5% and 97.5%).
References
Frank Bretz, Willi Maurer, Werner Brannath, Martin Posch: A graphical approach to sequentially rejective multiple test procedures. Statistics in Medicine 2009 vol. 28 issue 4 page 586-604. http://www.meduniwien.ac.at/fwf_adaptive/papers/bretz_2009_22.pdf
Author
Kornelius Rohmeyer rohmeyer@small-projects.de
Examples
est <- c("H1"=0.860382, "H2"=0.9161474, "H3"=0.9732953)
# Sample standard deviations:
ssd <- c("H1"=0.8759528, "H2"=1.291310, "H3"=0.8570892)
pval <- c(0.01260, 0.05154, 0.02124)/2
simConfint(BonferroniHolm(3), pvalues=pval,
confint=function(node, alpha) {
c(est[node]-qt(1-alpha,df=9)*ssd[node]/sqrt(10), Inf)
}, estimates=est, alpha=0.025, mu=0, alternative="greater")
#> lower bound estimate upper bound
#> H1 0.000000000 0.8603820 Inf
#> H2 -0.007600126 0.9161474 Inf
#> H3 0.000000000 0.9732953 Inf
# Note that the sample standard deviations in the following call
# will be calculated from the pvalues and estimates.
ci <- simConfint(BonferroniHolm(3), pvalues=pval,
confint="t", df=9, estimates=est, alpha=0.025, alternative="greater")
ci
#> lower bound estimate upper bound
#> [1,] 0.000000000 0.8603820 Inf
#> [2,] -0.007580967 0.9161474 Inf
#> [3,] 0.000000000 0.9732953 Inf
# plotSimCI(ci)